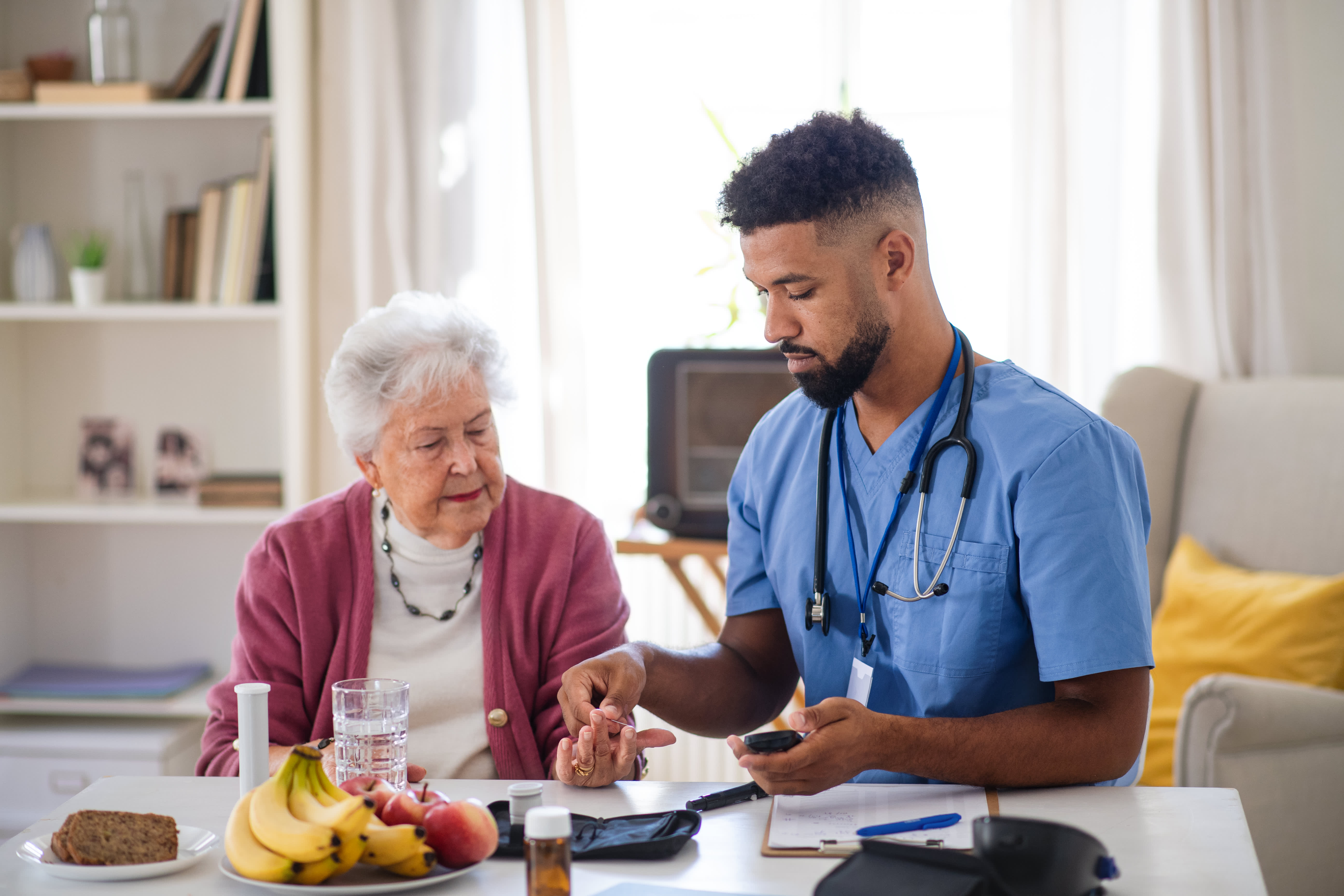
May 2025 CMS Policy Updates: What Payers and Providers Need to Know
Why May's Updates Demand Attention May 2025 marked a consequential month in U.S. healthcare policy. In a single wave of announcements, the Centers for Medicare & Medicaid Services (CMS) released new draft guidance for drug price...
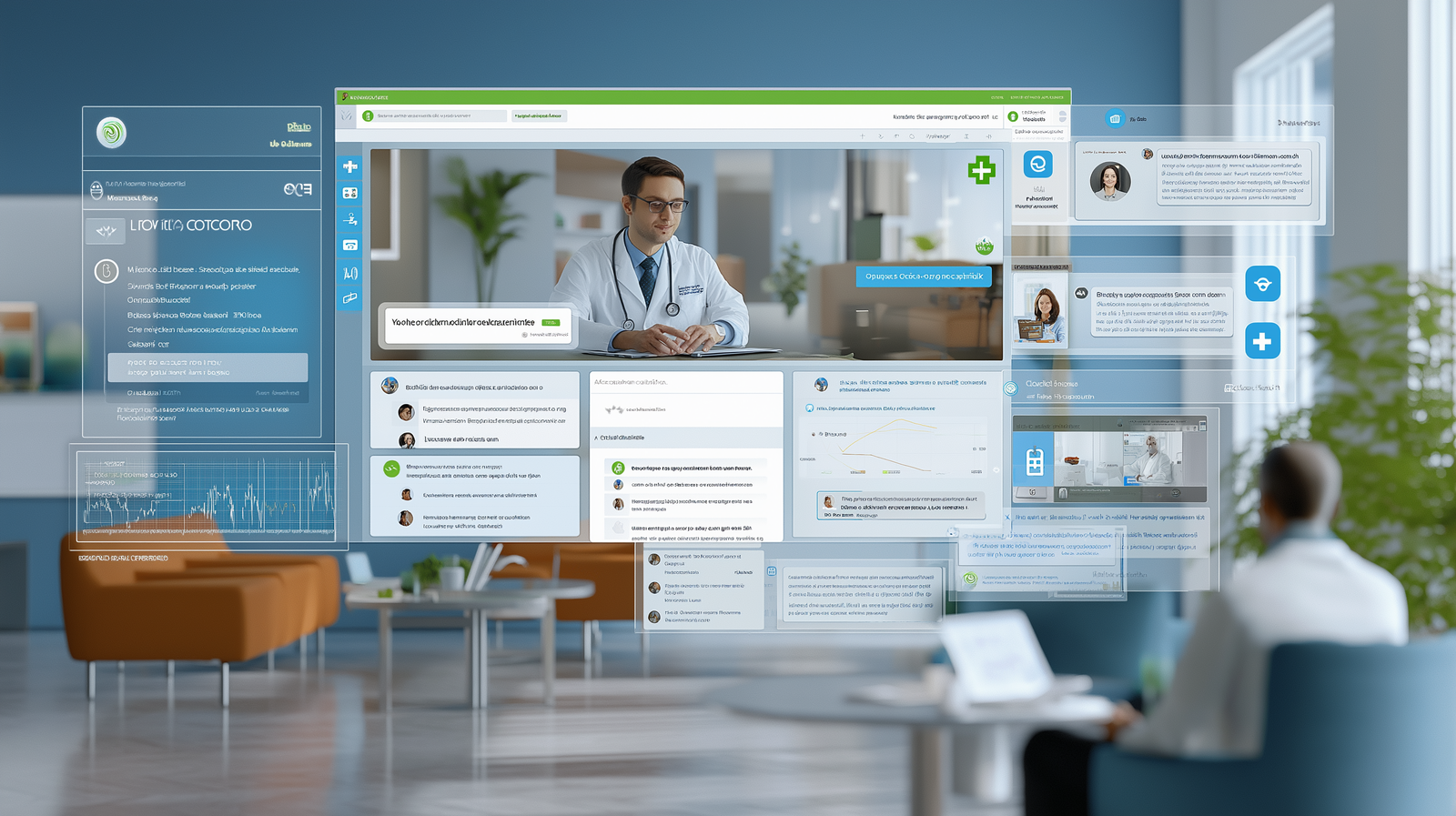
Fixing Prior Authorization: What the New CMS and HHS Pledge Means
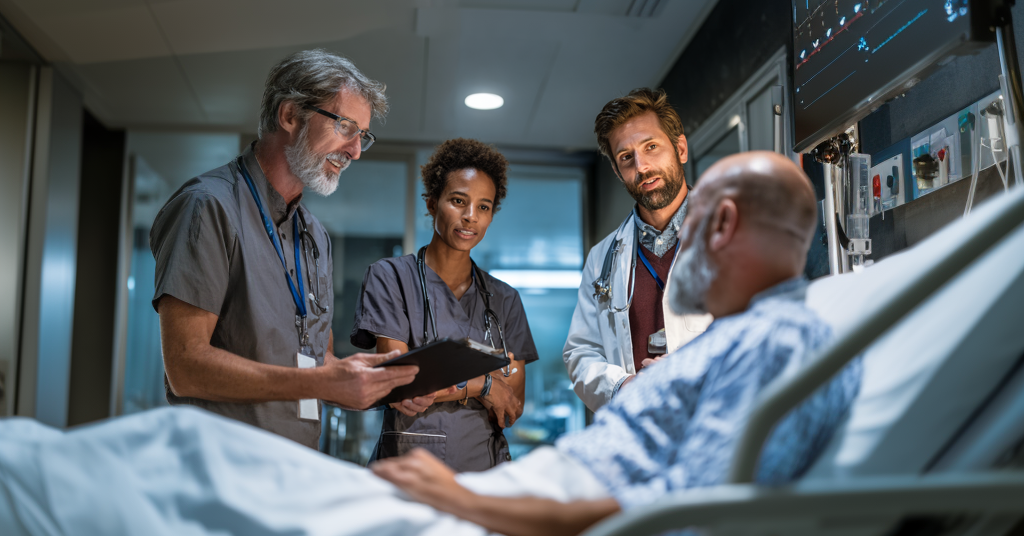
Using the Quintuple Aim to Shape an Effective AI Strategy in Healthcare
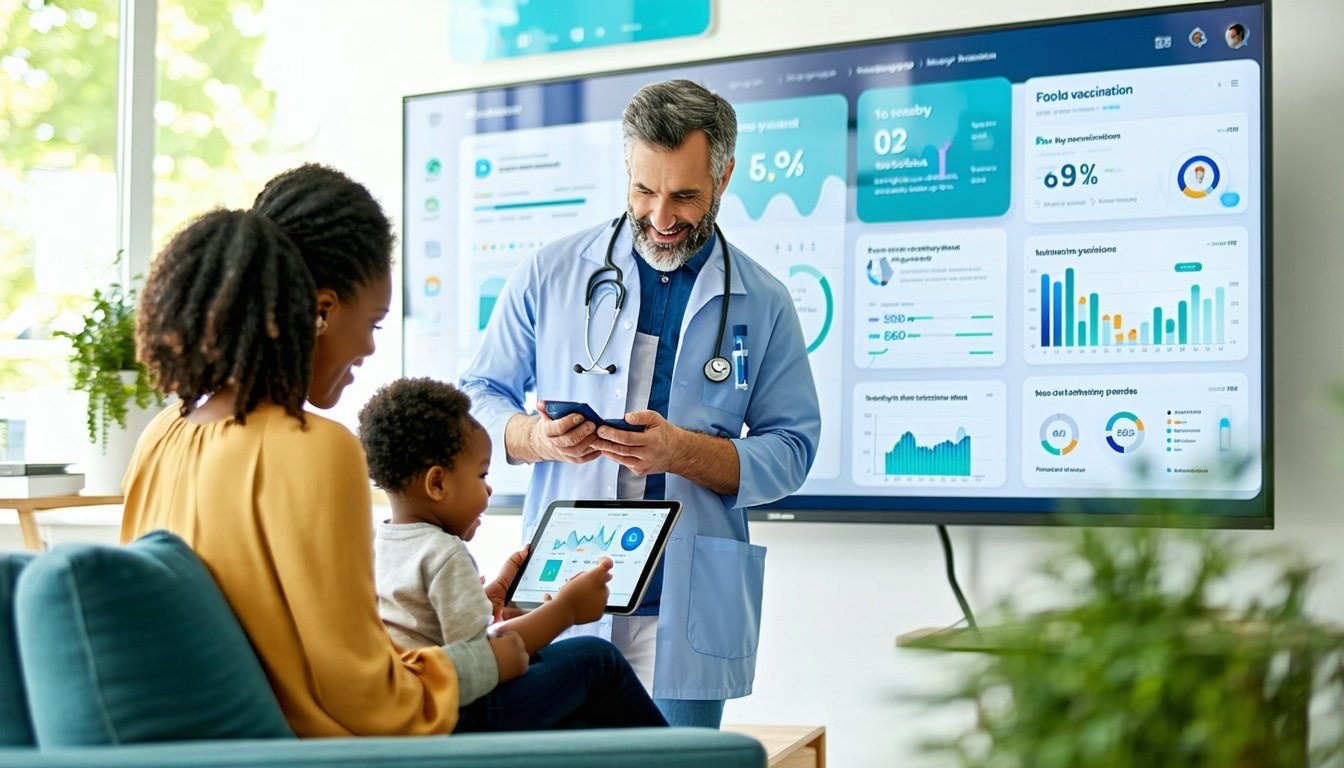
A Welcome Push Forward on Health Engagement
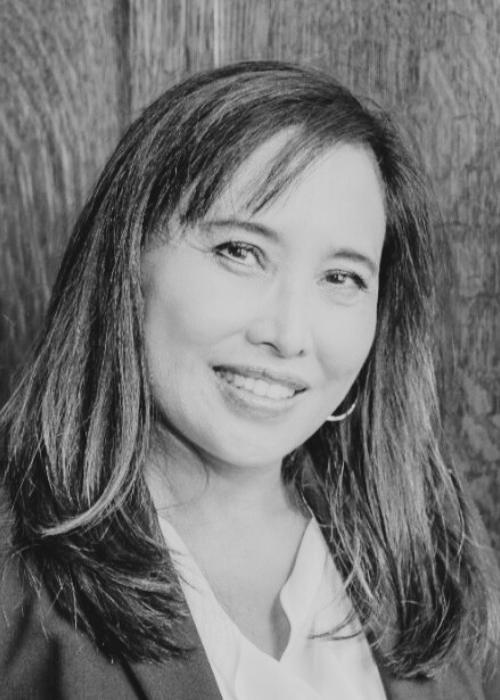
Season 2 / SITECORE
The changing role of the CMO in healthcare
Watch Video
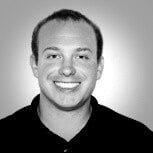
Season 2 / CENTRAK
Realtime location solutions for healthcare
Watch Video
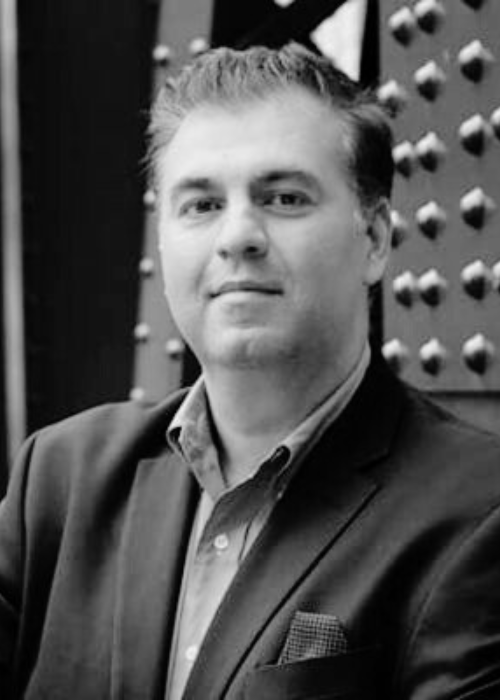
Season 2 / PE
Generative AI in healthcare: risks and rewards
Watch Video
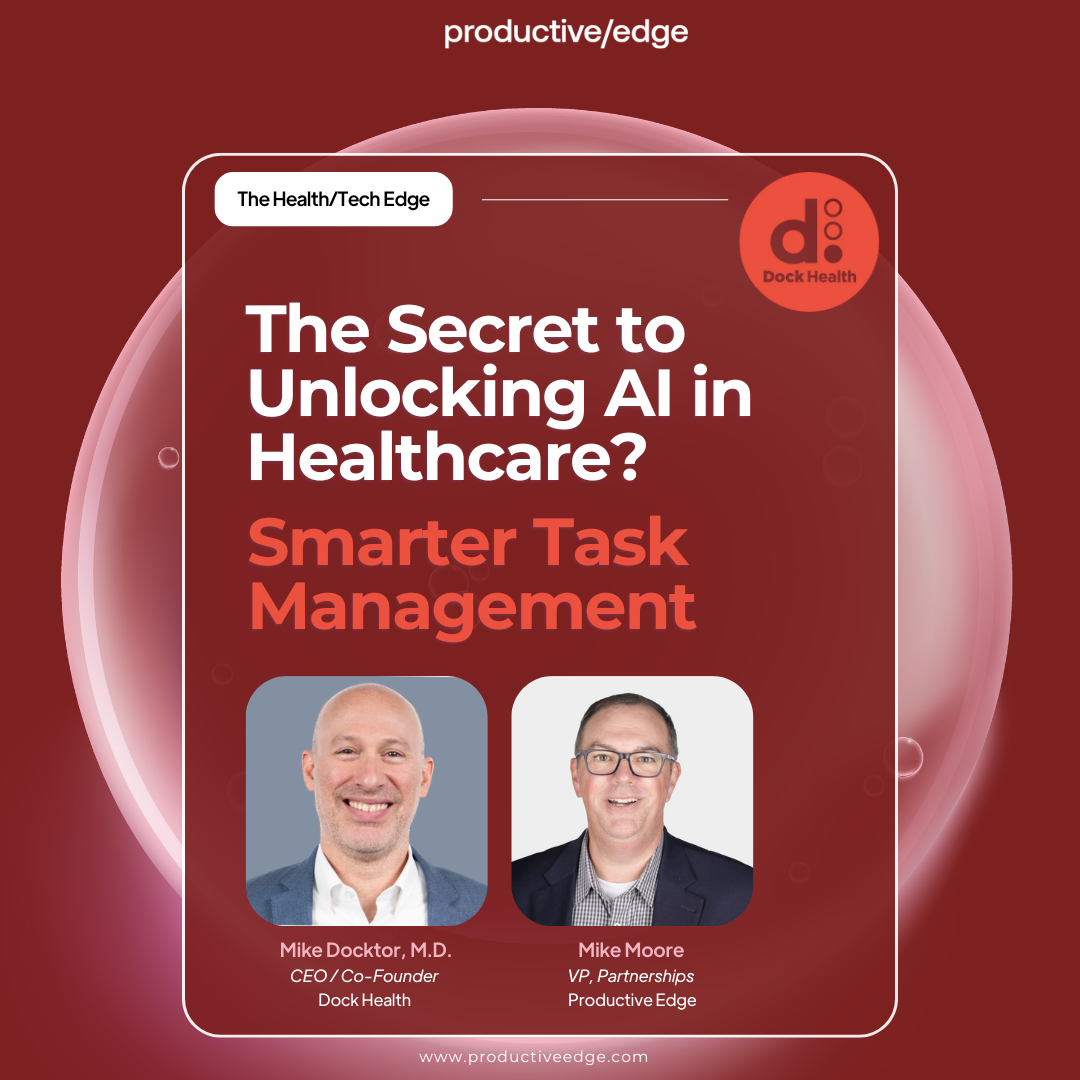
Unlocking AI in Healthcare with Smarter Task Management

How AI Agents Are Unlocking 24/7 Patient Support
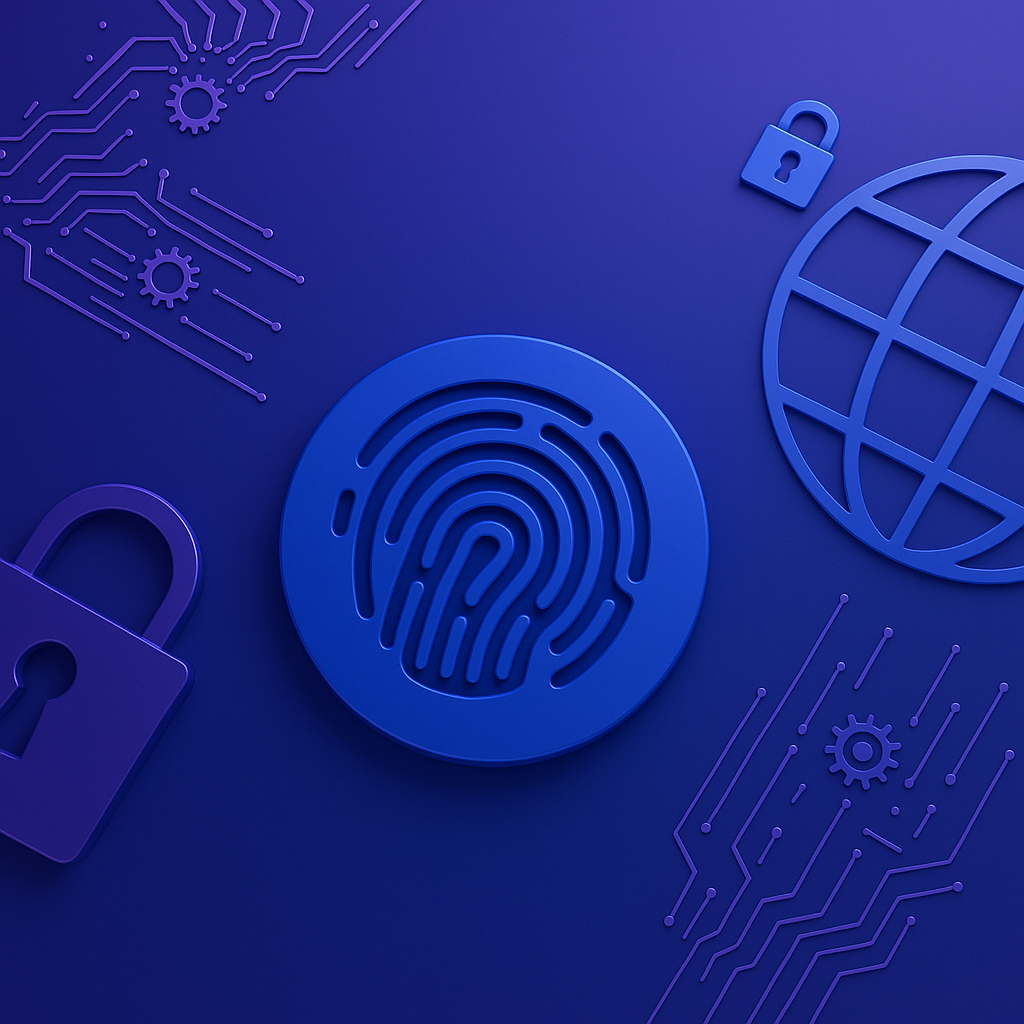
Governance, Compliance, and Risk Management for Healthcare AI Agents
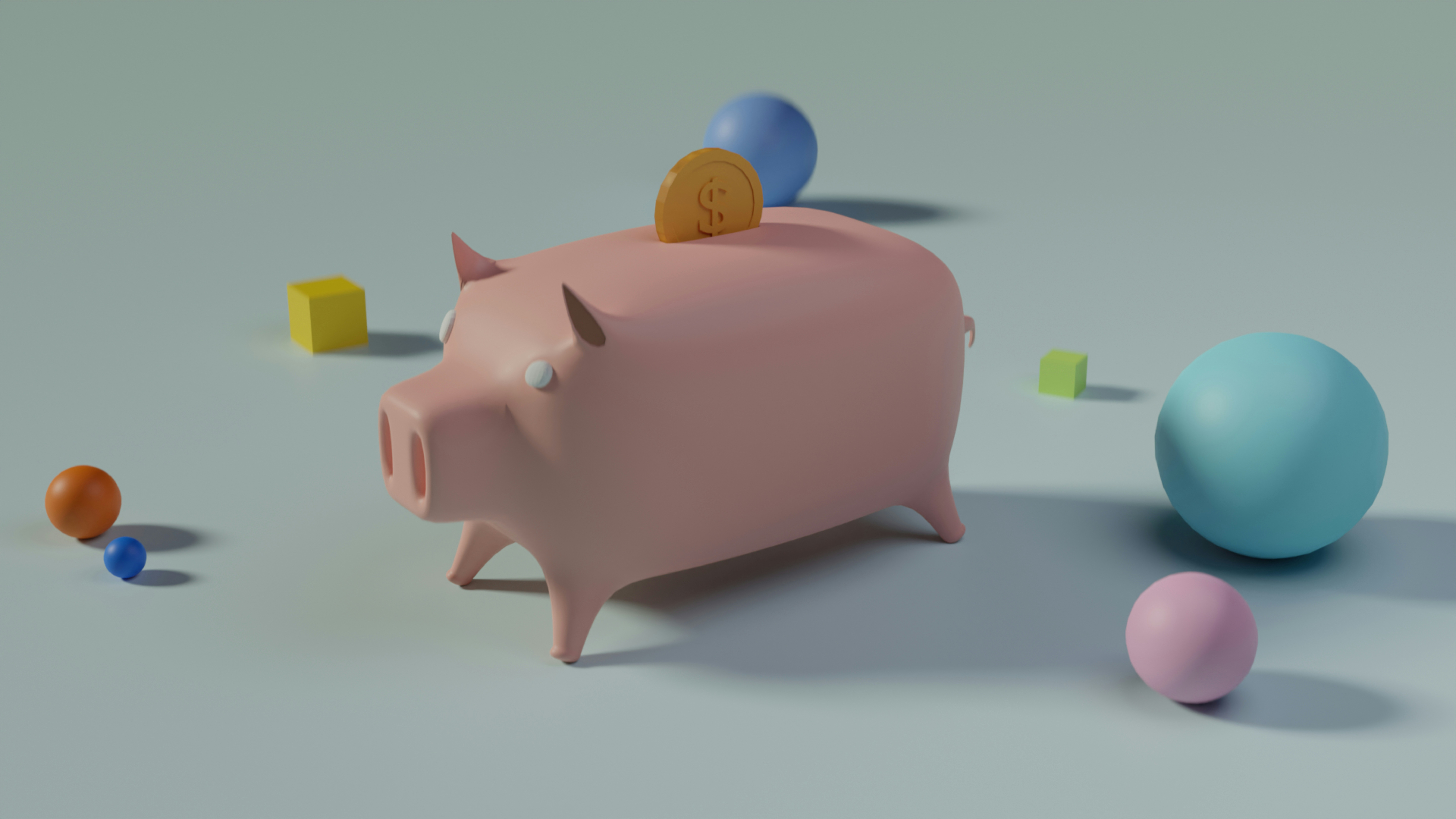
How AI is Helping Payers Recover Millions in Denied Claims
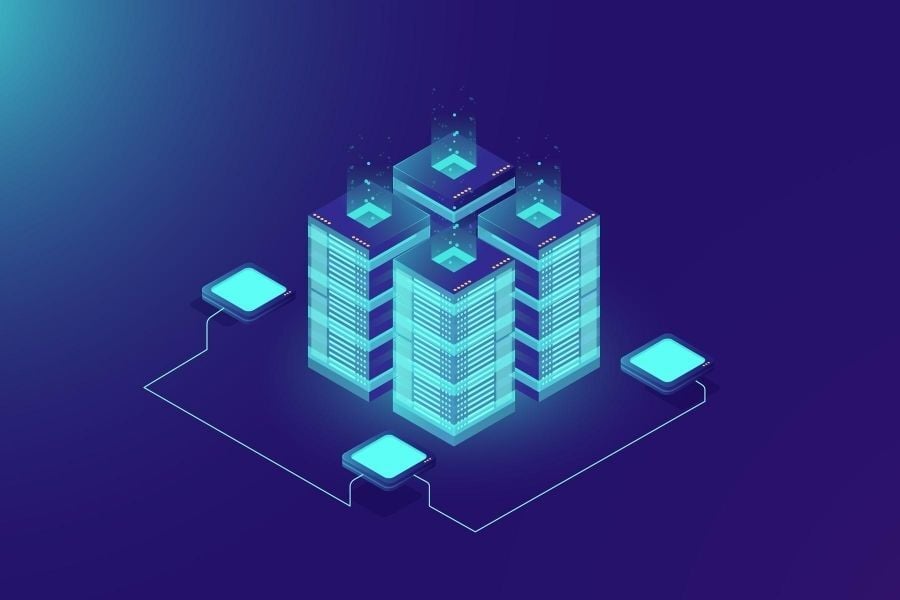
What is Microsoft Azure Data Lake and Why Healthcare IT Should Care
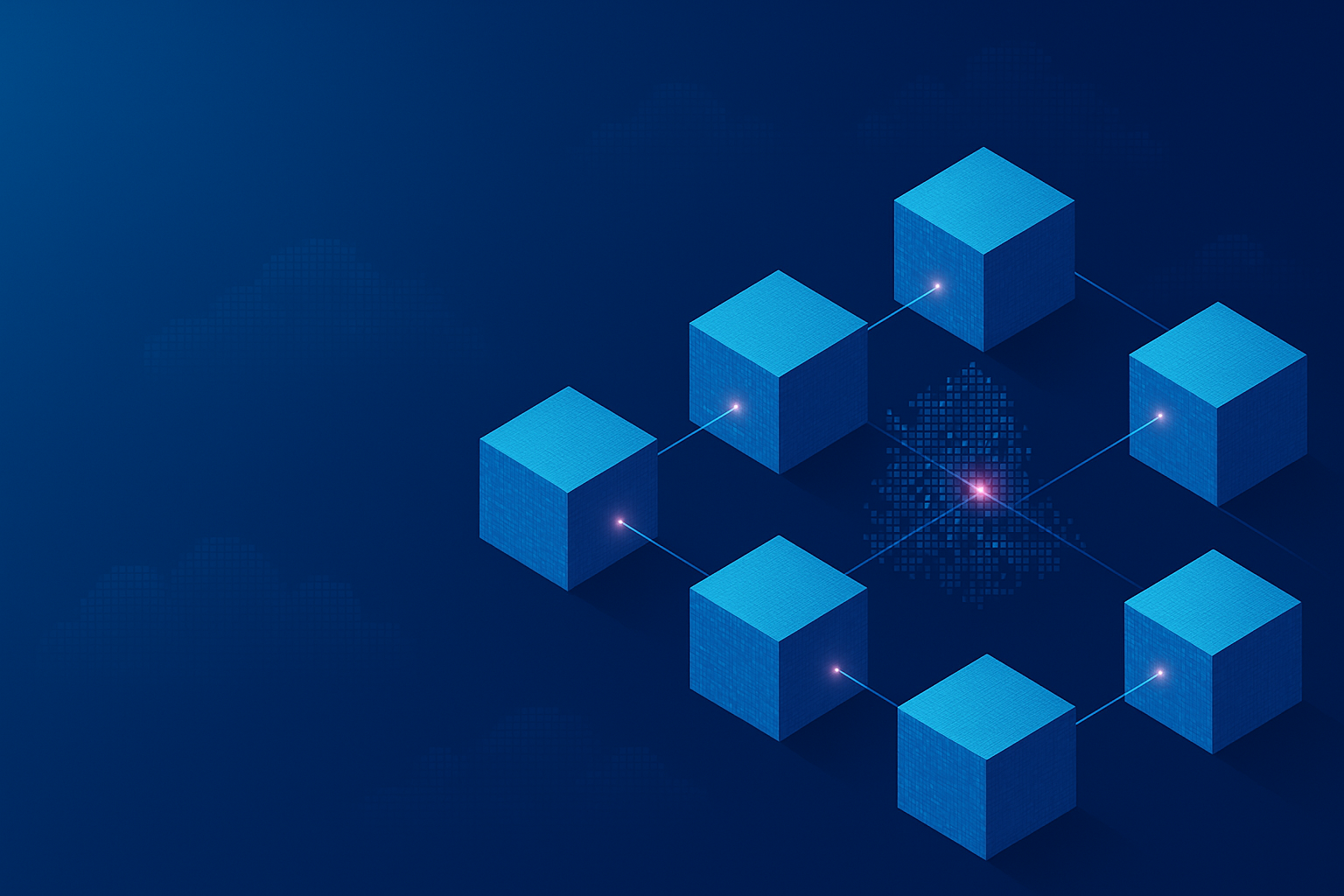